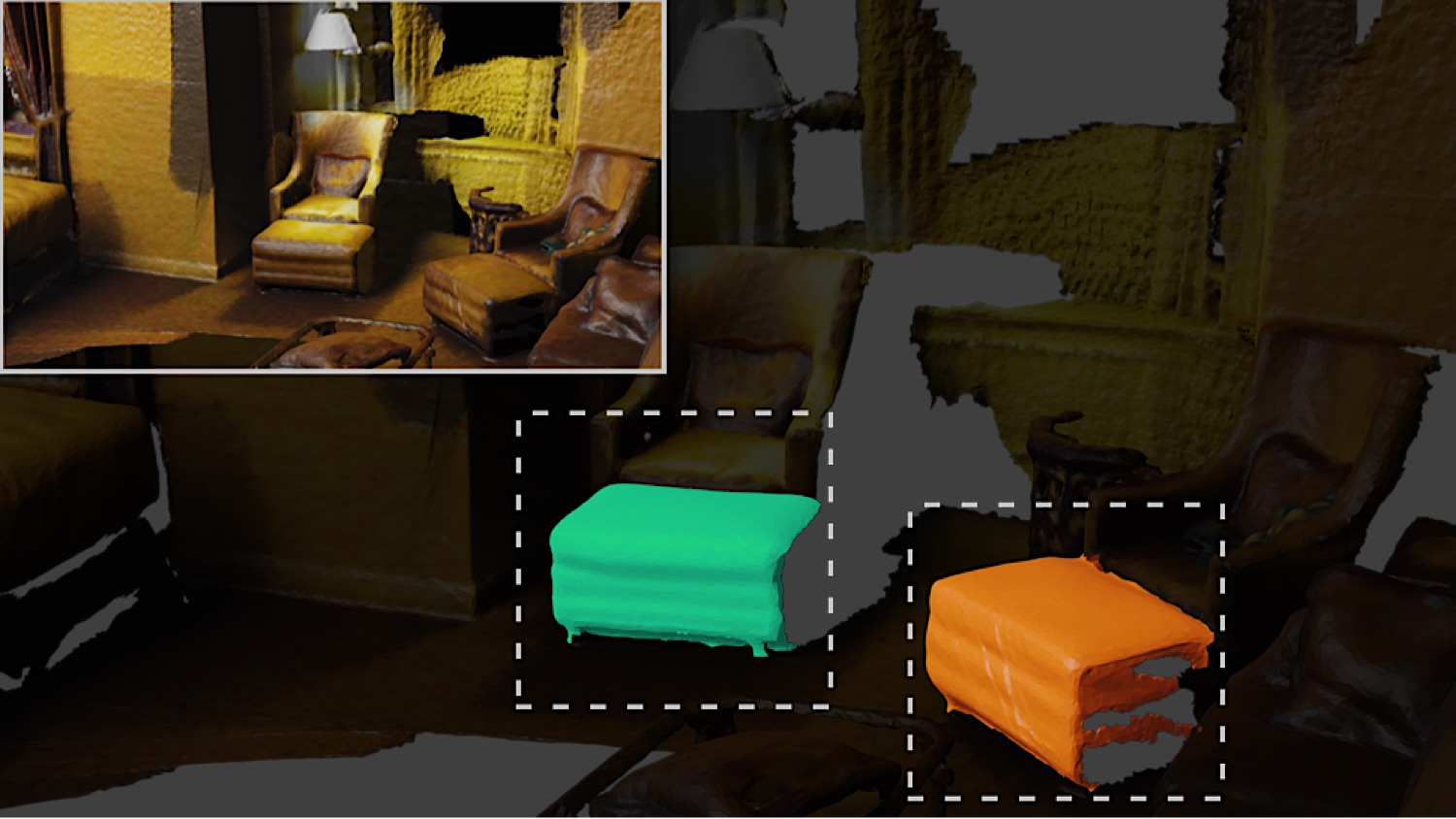
footrest
We introduce the task of open-vocabulary 3D instance segmentation. Current approaches for 3D instance segmentation can typically only recognize object categories from a pre-defined closed set of classes that are annotated in the training datasets. This results in important limitations for real-world applications where one might need to perform tasks guided by novel, open-vocabulary queries related to a wide variety of objects. Recently, open-vocabulary 3D scene understanding methods have emerged to address this problem by learning queryable features for each point in the scene. While such a representation can be directly employed to perform semantic segmentation, existing methods cannot separate multiple object instances. In this work, we address this limitation, and propose OpenMask3D, which is a zero-shot approach for open-vocabulary 3D instance segmentation. Guided by predicted class-agnostic 3D instance masks, our model aggregates per-mask features via multi-view fusion of CLIP-based image embeddings. Experiments and ablation studies on ScanNet200 and Replica show that OpenMask3D outperforms other open-vocabulary methods, especially on the long-tail distribution. Qualitative experiments further showcase OpenMask3D's ability to segment object properties based on free-form queries describing geometry, affordances, and materials.
Using OpenMask3D you can perform free form queries which capture uncommon objects, affordances, object properties, geometry, materials, and states.
footrest
angel
“sit”
“wash”
“an orange pillow”
“a yellow pillow”
“a circular table”
“a rectangular table”
“a leather chair”
“a fabric chair”
“a full garbage bin”
“an empty garbage bin”
@inproceedings{takmaz2023openmask3d,
title={{OpenMask3D: Open-Vocabulary 3D Instance Segmentation}},
author={Takmaz, Ay{\c{c}}a and Fedele, Elisabetta and Sumner, Robert W. and Pollefeys, Marc and Tombari, Federico and Engelmann, Francis},
booktitle={Advances in Neural Information Processing Systems (NeurIPS)},
year={2023}
}